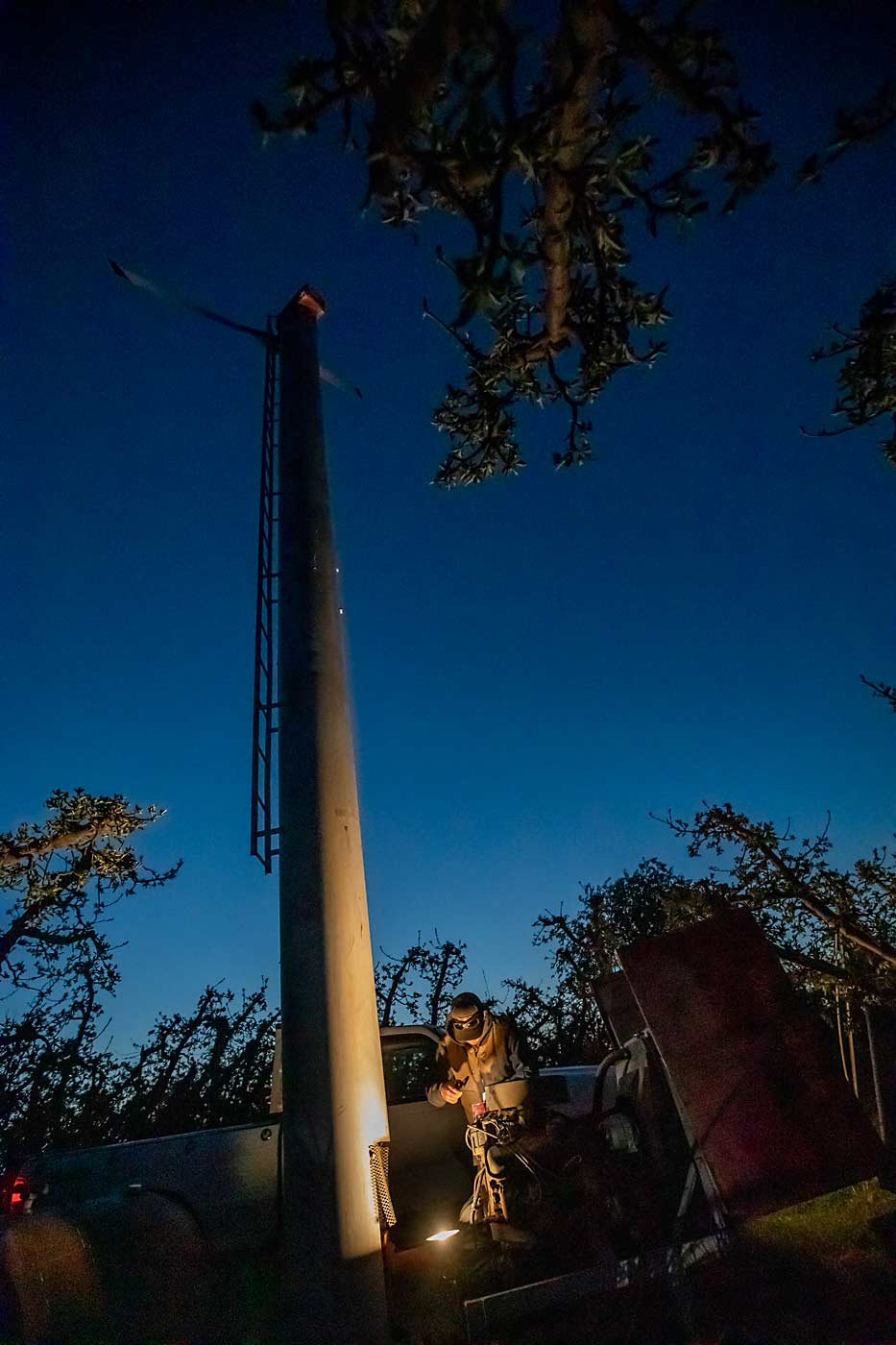
Conner Duim’s wind machines now start automatically when the temperatures dip toward bud-damaging thresholds, but the technology doesn’t mean he gets a good night’s sleep.
“When I was younger, my dad would religiously watch that Clearwest (frost forecast) report and the frost alarm would go off here at the house, and it would take him an hour to start all the wind machines,” said Duim, who farms with his father in the Yakima Valley. “Now, I just sleep with one eye open. I hear the wind machines start up and I’ll go drive around, making sure they are all running all right.”
Like his father and generations of growers before him, Duim relies on daily frost forecasts to know when he needs to go out and make sure everything is poised for frost protection.
“Having good forecasting is essential to having equipment up to date and running good,” he said. “And if it’s going to be warm, I can sleep well.”
For 101 years, nightly frost forecasts have helped Washington orchardists protect their crop, from lighting orchard heaters to tapping a smartphone to turn on wind machines or water today. While the technology of frost protection has advanced, the foundation of frost forecasting remains the same: comparing the existing weather conditions to similar patterns in the past to predict the likely lows.
“It’s all about the little microclimates. We’re dealing on a scale that is below what the forecast models are capable of doing,” said Bud Graves, who retired in 2020 from a meteorology career that started in 1970.
For years, he and longtime partner Jim Holcomb expected computer models — which greatly improved mid-range weather forecasts — to put them out of work. They founded Clearwest, a private forecasting company, to serve the tree fruit industry when the National Weather Service discontinued the practice in 1996. But by 2020, they were ready to retire, and the industry’s need remained, so they partnered with Washington State University’s AgWeatherNet to carry on the industry-supported work and keep the forecasts freely available to all growers.
While WSU meteorologists trained with Graves and Holcomb in 2020, WSU engineers began to train a computer model to do the same data-driven prediction process.
“If there is a way to beat a human forecaster, that’s the way to do it,” said meteorologist Craig Oswald, who worked on the transition from Clearwest to AgWeatherNet.
The regional forecast models that give most of us our weekly weather outlook lack the computational power to capture the microscale influences of terrain that make all the difference in orchard frost events, Oswald said. But with a machine-learning approach, a computer model can learn from all the recorded observations collected over the past 101 years, along with all the additional data that AgWeatherNet’s growing network of sensors now provides.
This approach replaces Graves and Holcomb’s decades of experience with computational power, Oswald said, but “it’s doing basically the same thing.”
“By applying simple machine learning, we are improving the forecast,” said Lav Khot, director of AgWeatherNet and an associate professor of agricultural systems engineering. He expects these algorithms to become the foundation of the frost forecasting next year.
Prediction patterns
When the U.S. Weather Bureau, which we now know as the National Weather Service, first set up frost districts in Yakima in 1922 and in Wenatchee in 1923, it installed observation stations around the regions, and collaborators would call in readings from minimum-temperature thermometers.
It took years to develop the climatology of frost risks, Holcomb said, getting to know cold air behavior on the landscape. From the start of his career in the 1960s until his retirement in 2020, he relied on the same forecasting approach, called analogy.
“We collected records of those cold nights and the weather patterns that existed and then looked at the current situations and tried to find a match that occurred in the past,” he said. “You would get a repeat of the same patterns.”
While computer models today learn those patterns rapidly from historical data, Holcomb and Graves recall learning from growers during many cold, dark nights.
“If you want to know the truth, the growers know more about the weather than we weathermen do,” Graves said. Early in his tenure in Hood River, Oregon, a grower pointed out to him that when Mount Hood was covered in clouds, he knew they would roll in and insulate the orchards.
Real-time weather sensors have changed how growers watch the weather, but not why.
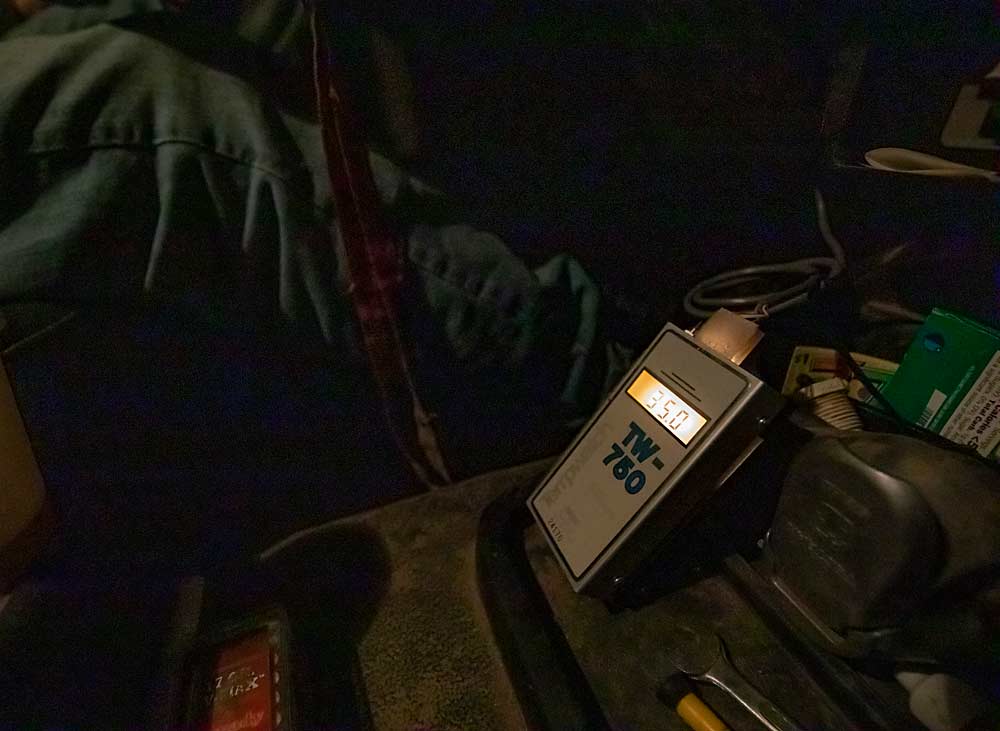
“Growers, they are watching the temperatures because their livelihoods are on the line,” Oswald said.
Growers report cold events to AgWeatherNet’s frost page, which helps forecasters check their work and continually improve.
“If you forecast 40 and raining, and it’s actually 44 and rainy, that doesn’t change anything for the average person, they are still going to need a raincoat,” Oswald said. “But if you are forecasting for frost, 4 degrees could be a big deal if you are right around the damage threshold for buds or flowers.”
The future of frost protection
Wind machines need inversions — warmer air layers floating above the settling cold — to pull down protective warmth into the orchard. To better document inversions, AgWeatherNet has installed 30 tall towers, known as mesonet towers, that have sensors at 2 meters and 9 meters. More are planned this year.
“This will certainly continue to help us understand microclimates,” said Nathan Santo Domingo, AgWeatherNet’s newest meteorologist.
On the research front, the machine-learning model is just one piece of a “field-specific, weather-driven, automated frost mitigation” project backed by $1 million from the U.S. Department of Agriculture. Led by Khot, the project includes inversion profile mapping of cherry orchards and blueberry fields, which is done by drones with thermal sensors.
The idea is to see how well the towers capture the inversion conditions at commercial locations several miles from weather towers and how much inversion variability exists across those farms.
Khot envisions the research helping growers understand where to place sensors that trigger the wind machines and identify sensors that best reflect the temperature the fruit buds experience — which can differ slightly from air temperatures.
“The drone data will tell you if your wind machine is doing what it is supposed to,” he said. “We want each grower to know their sites and know how the inversions behave.”
Another grant from the Washington State Department of Agriculture supports AgWeatherNet using machine learning to build station-specific air temperature and humidity forecasts for its entire network.
Santo Domingo encouraged growers to sign up — at no cost — to use AgWeatherNet’s tools and to send in their own reports.
“It’s a hyperlocal forecast. Having both the biological data and meteorological data, it really helps us narrow in on what producers need to know,” he said. “It’s a constant feedback loop, that’s one of the coolest things. It’s a huge collaborative effort to do this.”
—by Kate Prengaman
Kate,
Nicely done article on the frost forecasting program. Bud and I did frost forecasting for about 50 seasons, probably longer than any other forecaster in the USA. I think the forecast program is in good hands now at WSU Prosser.