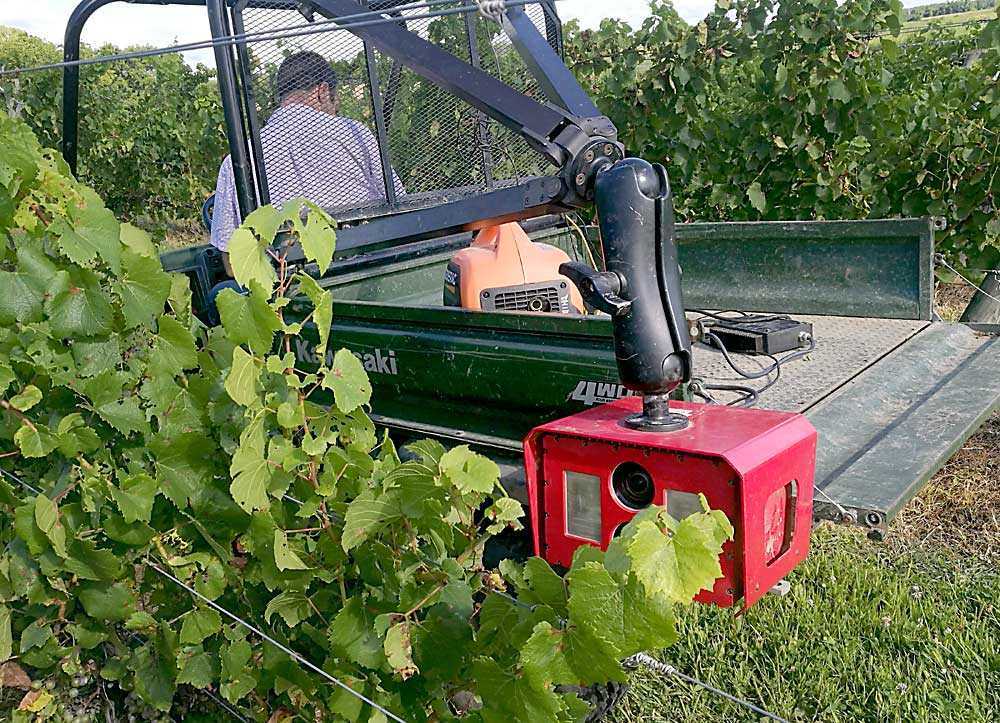
Artificial intelligence (AI) akin to that used in facial-recognition software is accelerating the grape-breeding process by accurately identifying those individual vines that carry favorable genetic characteristics, notably those that provide mildew resistance and higher fruit quality.
The robotic camera system developed at Cornell University, called Blackbird, could also help select parent breeding stock resistant to other pathogens and, as a more immediate benefit for growers, could be used to determine optimum fungicide combinations for different geographic localities.
Robotic camera
The Cornell-led, U.S. Department of Agriculture-funded VitisGen2 project uses a high-tech genetic sequencing approach, known as the rhAmpSeq system, to sift through the part of the genome that is common to all grapes and find DNA markers — bits of genetic code — that are associated with genetic traits of special interest to breeders.
Even with these advances, technicians still had to spend hours hunched over microscopes, manually scanning small circular leaf samples for signs of powdery and downy mildew infection. This entailed clearing away the chlorophyll from the leaf tissue, staining each leaf disk so the mildew’s filamentous and otherwise-transparent hyphae would show up, and assessing the presence and extent of the infection, said USDA research plant pathologist Lance Cadle-Davidson, who was part of the USDA-Cornell University team that developed rhAmpSeq for use on grape leaves.
The team, in collaboration with Printersys and the Mount Sinai Light and Health Research Center, has now developed the Blackbird robotic camera as a better way to do that task. The camera automatically scans the leaf disks, using ideal lighting to see the mildew with no need to stop the infection or stain the leaf tissue.
“By automating it, what once took two months of a technician at a microscope, now we can do in one day,” Cadle-Davidson said. “So, this is a huge increase in our throughput and our capabilities and allows us to do experiments that we couldn’t even envision before.”
One capability is what he calls live imaging, which permits them to observe a leaf disk over several days. “That is really powerful for scientific research where we want to track the dynamics of the infection process over time,” he said. The researchers have also begun testing how resistance genes respond to different strains of fungus, as a way to predict how the fungus might evolve to overcome those genes. Such understanding, he said, “would really guide the strategies for the breeders and how they develop resistant varieties.”
Trained AI
The next step was to get as much biological information as they could from the images, and for that, Cornell engineer and computer scientist Yu Jiang stepped in. An assistant research professor of systems engineering and data analytics for specialty crops, Jiang took a cue from facial recognition software that scans and matches facial features and got to work on an AI algorithm that could recognize the spores and fine threads, or hyphae, that make up powdery and downy mildews.
Technicians in Cadle-Davidson’s group provided the AI’s training materials, which included one more tedious task: looking at one leaf disk after another and noting where they saw the tiny hyphae on each one. Jiang and his group made the job as straightforward as possible: They instructed them to look at miniscule sections of each leaf disk — rather than the whole thing at once — and note it with a simple “yes” if hyphae were present, or “no” if they weren’t. By feeding those yes/no notes on each tiny leaf-disk section into the AI algorithm, it learned to identify which were infected and which were not.
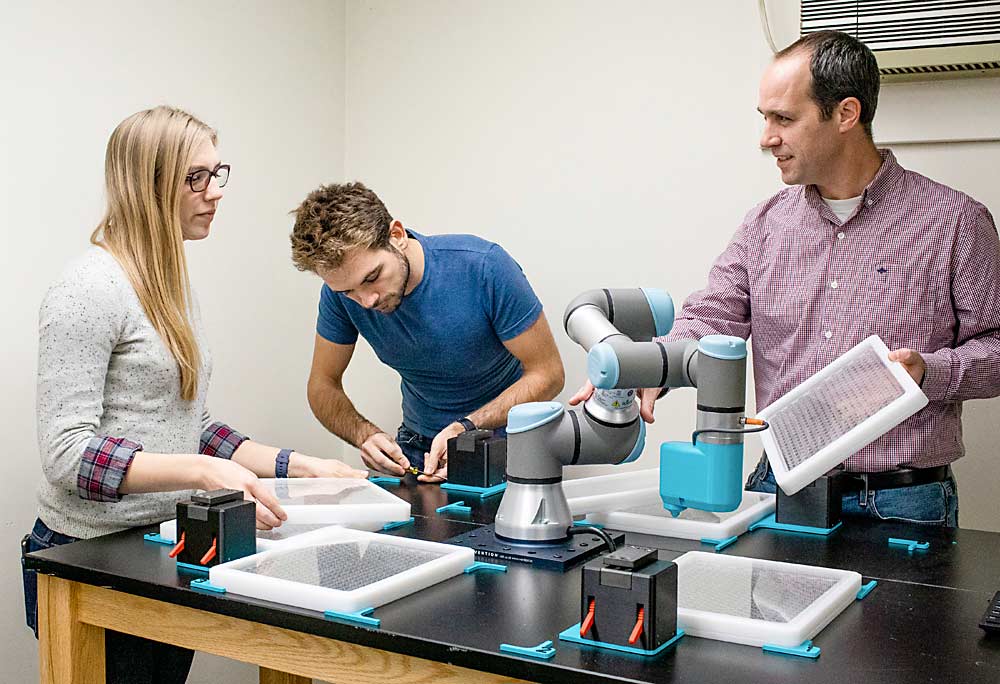
It not only worked, but worked exceptionally well, Jiang said. “We have already found that this method sometimes can even outperform human experts in terms of the quantification accuracy,” he said.
Jiang also envisions deploying the AI-assisted robotic camera technology as a way to test how well chemical fungicides perform against mildews. He has begun working with Cornell grape pathologist Kaitlin Gold to use this system in concert with other research tools to determine the efficacy of different fungicide combinations for various localities in New York state.
“We hope to transfer this knowledge to our local growers, and that will have some immediate benefits,” Jiang said.
Digital revolution
Jiang now hopes to advance the AI-assisted camera system by switching out the standard color camera in Blackbird for a hyperspectral version that can see light beyond the range visible to the human eye. These additional wavelengths of light reveal so-called spectral signatures of additional chemical compounds, which in turn will provide insights into the interaction between the pathogens and leaves, as well as the differences between pathogen isolates (variants), some of which may be more harmful than others, he said.
Cadle-Davidson and Jiang are also collaborating on another project to design a camera-AI system to be used in the field.
“Our next step, and something we’ve been working on for the past three years now, is imaging in vineyards, so trying different types of sensors to develop the artificial intelligence algorithms to detect diseases,” Cadle-Davidson said. “Yu’s group has already done a phenomenal job of detecting and quantifying downy mildew in vineyards — it’s really beautiful — and now we’re working on powdery mildew.”
Potentially, such a system could be attached to a tractor and snap images of every vine in the vineyard, he said. And through a new USDA-ARS grant, the Cornell-USDA team is also now looking into expanding the Blackbird-AI system’s applications to other crops, pests, diseases and traits (see “Breeding insights”).
“I think AI will be the technology that really revolutionizes almost every single aspect in our daily lives,” Jiang said, noting that the same cutting-edge advances that are transforming medicine, aeronautics and other fields are also transforming agriculture. “That’s why we call this a new revolution of agriculture: a digital revolution.”
—by Leslie Mertz
Leave A Comment